Few retailers need to be convinced about the power quality data can have in making informed business decisions. Yet, a recent global survey by Forrester Consulting and WNS found that, while 63% of business leaders recognise that data and analytics are strategic enablers, just 37% have prioritised investments in these capabilities.
The issue is especially acute within the retail and CPG sectors, where 73% of brands with strong data maturity have achieved quantifiable business value as a result. The kicker is, according to the findings from the Capgemini Research Institute, just 6% of retailers clear the benchmark for becoming “data masters” in the first place.
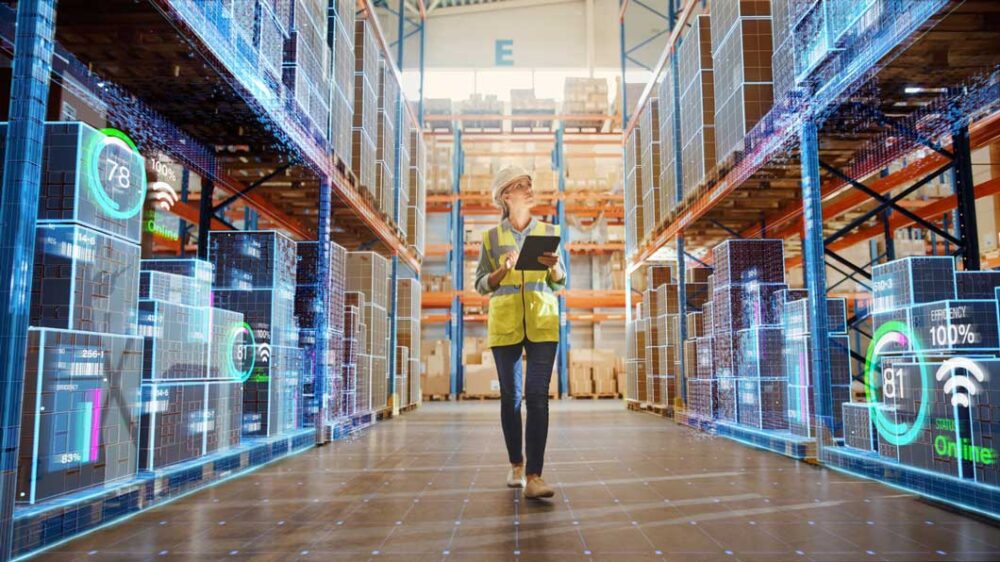
Those in the success bracket include Berlin-based food service Delivery Hero, which uses data points around “customer lifetime value” as a core metric to drive strategic decisions, such as whether or not to enter a new market. Likewise, veteran suit maker Moss Bros, which recently enjoyed a post-Covid rebound fuelled in part by its laser-like focus on data optimisation. The fashion label uses a combination of advanced intelligence, A/B testing and biometrics to analyse customer behaviours; shaping an in-depth understanding of what visitors want, and where exactly their pain points lie.
So how can other brands follow suit, smoothly transitioning from raw to enriched data intelligence? Here are four key steps:
1. Identify a starting point
There are all sorts of frameworks used to measure data maturity. Still, generally, retailers will fall on a scale from ad hoc – where data is used in a piecemeal and fragmented way – to data-guided, whereby data has a clear purpose and drives decisions across the organisation.
In-between stages on this spectrum include siloed – where data exists and is regularly collected, but is not easily accessible – and embedded, where data is centralised and drives decision-making, but may not be completely clean.
The epitome of data maturity in retail is to be data-guided, where all the available information is being used to inform business direction, and possibly even inspire new growth or revenue streams.
For example, pioneering smart speaker brand Sonos uses a “test and learn” data culture to constantly iterate new ways of evolving its market presence across 50+ streaming services in dozens of countries worldwide. This involves bringing together insights from first and third-party data, consumer research, retail and sales trends, its product roadmap and more, as well as experimenting with new approaches to optimise these learnings.
2. Cleanse your data
The cleaner the data, the more accurate and trustworthy it is. Data that’s been consolidated after spending most of its life in silos usually requires some cleansing. To ensure the data is able to layer properly and provides the most valuable insights possible, it’s also worth creating consistency in defining and measuring specific data points.
Brazilian retailer, Lojas Americanas, distributes 137 million items from over 120,000 third-party e-commerce vendors, with 41,000 staff. To ensure 100% accuracy and real-time monitoring for its internal systems at such a large scale, the e-commerce giant recently introduced a new system of machine-supported data cleansing. The automated data quality filter is designed to improve operations and customer experience across a vast network of interactions.
But does data always need to be squeaky clean in this way? It depends on its purpose. A retailer aiming to incorporate a Customer Data Platform (CDP) for personalisation and segmentation purposes could do so at the embedded stage. However, those intending to use their CDP to gain a full 360-view of their customer across multiple channels should aim to be data-guided.
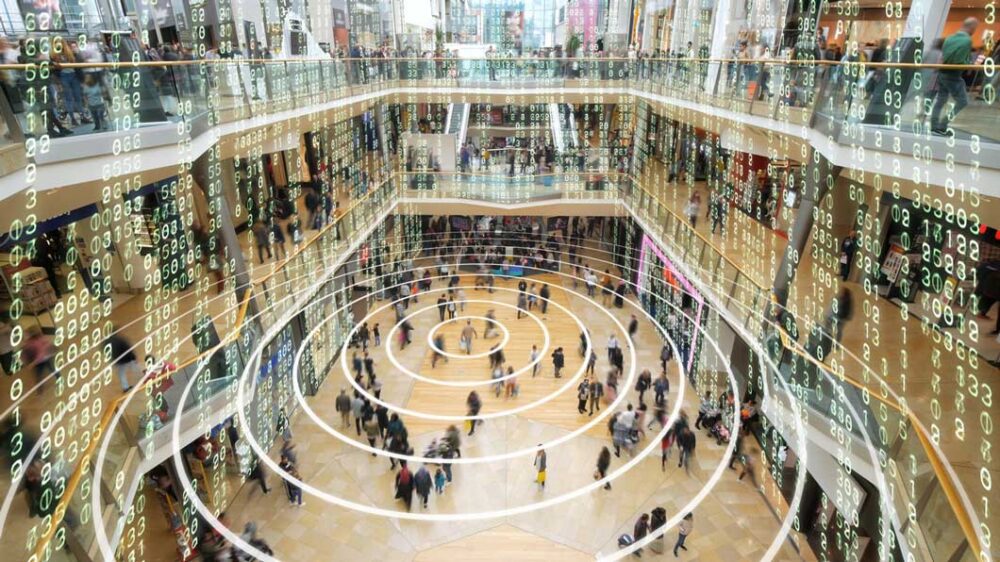
3. Adopt a people-first approach
While data generally appears as numbers, it carries a strong human element; it’s really about people. So, when embarking on a data transformation, it’s crucial that retailers gauge the level of receptiveness to data sharing between teams.
Key questions to consider include whether your team is ready for change, what level of executive support you have, if specialist training is required, and where areas of resistance or risk may crop up. It’s also useful to undertake a frank assessment of your current data culture, and identify which individuals or departments stand out as champions of change.
Online florist Bloom & Wild, for example, uses data wherever there is an opportunity to improve its customer’s “full lifecycle experience”. Pulling out meaningful trends across marketing spend, onsite behaviour, warehouse data and more, it has worked hard to involve its entire brand in the decision-making process amid rapid growth. The company’s director of business intelligence and analytics, Mairead Masterson, says it has explored how stakeholders use data, and how it standardises definitions, so that everyone has “a common language about data and why it is important”.
The aim is to have data that presents valuable insights to all users. To effectively achieve that, retailers need to keep their internal teams front of mind.
4. Build monetisation strategies
While meaningful insights are certainly beneficial, retailers who have reached the level of being data-guided can unlock additional value by monetising their data.
Data monetisation requires the leveraging of clear, reliable metrics, and it typically takes two forms. Indirect monetisation uses data to drive engagement and offers information that keeps users logging into the digital product. This approach can help an app find a foothold with its user base during those crucial first few months.
Direct monetisation uses existing data to create an entirely new revenue stream, perhaps as a premium subscription, API access or data-as-a-service offering. Styling service Stitch Fix, for example, uses its extensive data to offer real-time personalisation for customers (based on style, body shape, budget and more) via its e-commerce website, Freestyle. It also recently used 75 million data points from its customers buying plus-size clothing to create a plus-size toolkit that it shares with partner brands, helping them make better merchandising decisions.
In Conclusion
The journey to becoming data-driven is filled with obstacles. The rewards of transitioning, however, are huge. Levi Strauss and Co. is one of a growing cohort of retailers now looking to use digital selling data to manufacture more precisely, based on consumer demands. As its executives point out, this reversal of the traditional manufacture-to-sell life cycle has huge implications for creativity, sustainability and the bottom line.
Keeping in mind the above essential elements, retailers can be well on their way to doing the same. By breaking down silos, they have the potential to reshape their entire culture; creating tangible value, and revenue streams fit for a data-first age.